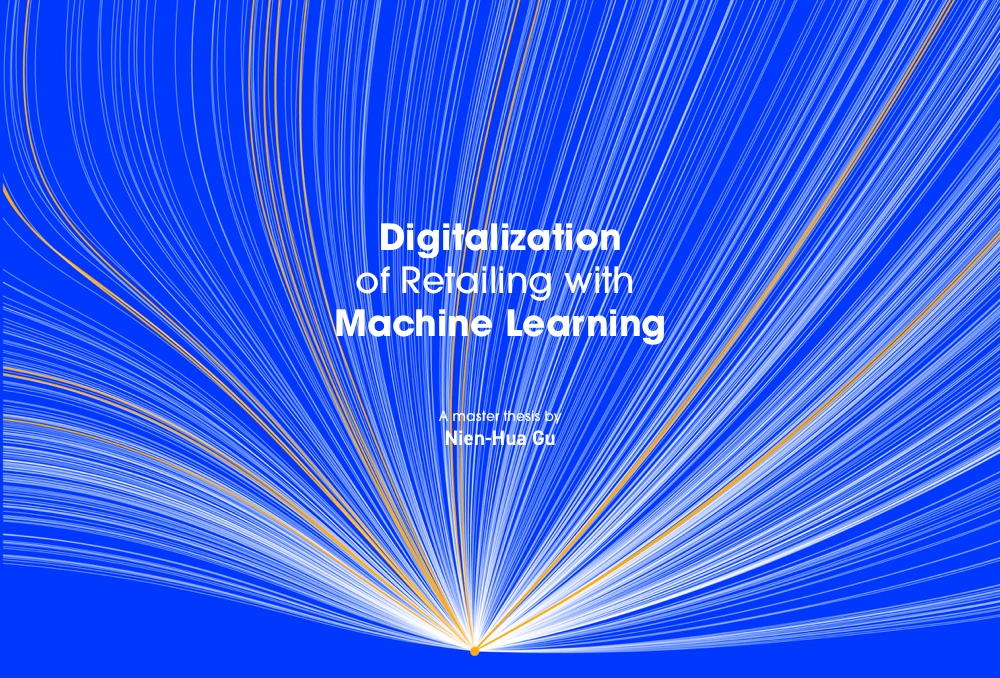
Digitalization of Retailing
A Service-Product Design for Digital Merchandisers to Implement Machine Learning
What are the possible implementation strategies of Machine Learning? And how could designers facilitate digital retailers to do it?
It is my master thesis that will be published in late August.
A collaborative project with

Introduction
This thesis is executed in collaboration with BloomReach. This thesis focuses on the implementation issues of Machine Learning for retailers. The aim is to improve BloomReach’s post-sales services and products to facilitate their customers from digital retail industry to implement Machine Learning technology in their service.
Machine Learning is a state-of-art technology for digital retail companies. This technology is proven to be beneficial for both users and companies, providing personalized user experience and improving the sales numbers of companies (Accenture, 2017). Digital disrupters win the market by this technology, such as Google (Davidson et al, 2010), Spotify (Bernhardsson, 2009) and Amazon (Linden, 2003). Threatened by the facts, digital retailers start to implement this technology by collaborating with technological solution providers. It is estimated that 90% of companies will rely on solution providers to design, build and implement digital technologies including Machine Learning by 2021 (Guseva et al, 2019). Therefore, Machine Learning becomes a key component to compete in the digital retail industry, and a viable business for digital solution providers.
The context of the project is based on the business-to-business service of BloomReach - Search & Merchandising (brSM) - a Machine-Learning-powered recommendation system. It enables the digital retailers to customize the personalized contents, recommendations and search results for their customers. brSM consists of digital software in the Platform-as-a-Service (PaaS) model and the in-person services (Figure 1). BloomReach provides services to build the service and train the business clients, and then the clients use the software to perform the optimization and generate more revenue.
However, digital retailers have challenges to implement Machine Learning. In a survey of executives aware smart technologies in the U.S., 47% of managers have difficulties to integrate the technology with existing process and system (Davenport & Ronanki, 2018). For the retailers, specifically, due to the inflexible company structure and slow decision making, they fail to incorporate Machine Learning into their value chain (Baird, 2018). Furthermore, Machine Learning requires several data-driven skills and methods to be fully implemented. The retailers need to perform iterative optimizations to unleash its full potential. Due to the statistic model that Machine Learning applies, it requires a data science method with a well-formulated structure and iterative process. Additionally, analytical skills, technical skills and effective performance metrics are required (Mulhern, 2009). They need to learn the use of telemetry data and A/B test technique before they can optimise the algorithm (Yang et al, 2018b). In order to help the companies to implement Machine Learning, some new strategic frameworks are invested (Davenport & Ronanki, 2018; Ng, 2018; Shankar, 2018). But it is unclear how these frameworks will be applied to the real company setting and how they will influence Machine Learning.
It is, therefore, crucial for a service provider like BloomReach to understand how digital retailers implement the technology and what difficulties they encounter. Doing so can positively impact the adoption rate of the service by their customers. This can also help the company to improve its current service and product from user perspective.